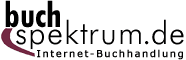 Neuerscheinungen 2018Stand: 2020-02-01 |
Schnellsuche
ISBN/Stichwort/Autor
|
Herderstraße 10 10625 Berlin Tel.: 030 315 714 16 Fax 030 315 714 14 info@buchspektrum.de |
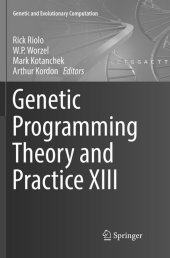
Arthur Kordon, Mark Kotanchek, Rick Riolo, W. P. Worzel
(Beteiligte)
Genetic Programming Theory and Practice XIII
Herausgegeben von Riolo, Rick; Worzel, W. P.; Kotanchek, Mark; Kordon, Arthur
Softcover reprint of the original 1st ed. 2016. 2018. xx, 262 S. 38 SW-Abb., 31 Farbabb. 235 mm
Verlag/Jahr: SPRINGER, BERLIN; SPRINGER INTERNATIONAL PUBLISHING 2018
ISBN: 3-319-81706-X (331981706X)
Neue ISBN: 978-3-319-81706-4 (9783319817064)
Preis und Lieferzeit: Bitte klicken
These contributions, written by the foremost international researchers and practitioners of Genetic Programming (GP), explore the synergy between theoretical and empirical results on real-world problems, producing a comprehensive view of the state of the art in GP. Topics in this volume include: multi-objective genetic programming, learning heuristics, Kaizen programming, Evolution of Everything (EvE), lexicase selection, behavioral program synthesis, symbolic regression with noisy training data, graph databases, and multidimensional clustering. It also covers several chapters on best practices and lesson learned from hands-on experience. Additional application areas include financial operations, genetic analysis, and predicting product choice. Readers will discover large-scale, real-world applications of GP to a variety of problem domains via in-depth presentations of the latest and most significant results.
Evolving Simple Symbolic Regression Models by Multi-objective Genetic Programming.- Learning Heuristics for Mining RNA Sequence-Structure Motifs.- Kaizen Programming for Feature Construction for Classification.- GP as if You Meant It: An Exercise for Mindful Practice.- nPool: Massively Distributed Simultaneous Evolution and Cross-Validation in EC-Star.- Highly Accurate Symbolic Regression with Noisy Training Data.- Using Genetic Programming for Data Science: Lessons Learned.- The Evolution of Everything (EvE) and Genetic Programming.- Lexicase selection for program synthesis: a Diversity Analysis.- Using Graph Databases to Explore the Dynamics of Genetic Programming Runs.- Predicting Product Choice with Symbolic Regression and Classification.- Multiclass Classification Through Multidimensional Clustering.- Prime-Time: Symbolic Regression takes its place in the Real World.